In-Process Components - Case Study of Flexible Digital Detector Arrays
Tracks
Energy
Tuesday, October 22, 2024 |
2:00 PM - 2:30 PM |
206 - Short Course & Technical Session |
Details
ASME NDE code BPVC.V-2023 and other radiography standards require that a single wall exposure technique be utilized “whenever practical.” This makes the potential application of examining curved parts using flexible digital detector arrays (DDAs) compelling. Flexible DDAs allow for digital images to be created using a single wall exposure technique much faster than by using computed radiography (CR) or film. In addition to use for examining pre-existing targets, such as pipelines, flexible DDAs can also be applied to evaluating components as they are in development. Flexible DDAs have been taken into manufacturing facilities and tested with different styles of parts.
Speaker
Katie Carpenter
NDT Imaging Scientist
Carestream NDT
In-Process Components - Case Study of Flexible Digital Detector Arrays
Presentation Description
ASME NDE code BPVC.V-2023 and other radiography standards require that a single wall exposure technique be utilized “whenever practical.” This makes the potential application of examining curved parts using flexible digital detector arrays (DDAs) compelling. Flexible DDAs allow for digital images to be created using a single wall exposure technique much faster than by using computed radiography (CR) or film. In addition to use for examining pre-existing targets, such as pipelines, flexible DDAs can also be applied to evaluating components as they are in development. Flexible DDAs have been taken into manufacturing facilities and tested with different styles of parts.
Biography
Katie is an imaging scientist working on industrial digital and computed radiography systems and is pursuing a level 3 radiography certification. Katie is involved with the ASTM radiography standards committee as the secretary for the ASTM E07.01 NDT subcommittee on radiography. She is also a member of the ASNT committee on artificial intelligence and machine learning and is a member of the ASNT engineering council AI/ML committee. Katie is a graduate of Rochester Institute of Technology with a doctorate in color science and of SUNY Oneonta with a bachelor’s degree in physics.
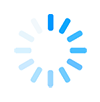